Meta’s Brain-to-Text AI Technology: Brain2Qwerty
Meta AI has unveiled Brain2Qwerty, a groundbreaking non-invasive brain-to-text decoding system. This innovative technology leverages magnetoencephalography (MEG) and electroencephalography (EEG) to translate brain signals into text, opening up new possibilities for individuals with speech or motor impairments. In this article, we’ll dive deep into how Brain2Qwerty works, its remarkable features, challenges, and future potential.
Table of Contents
- Introduction to Brain2Qwerty
- How Brain2Qwerty Works
- Key Features of Brain2Qwerty
- Performance Metrics
- Challenges and Limitations
- Future Directions
- FAQ
- Conclusion
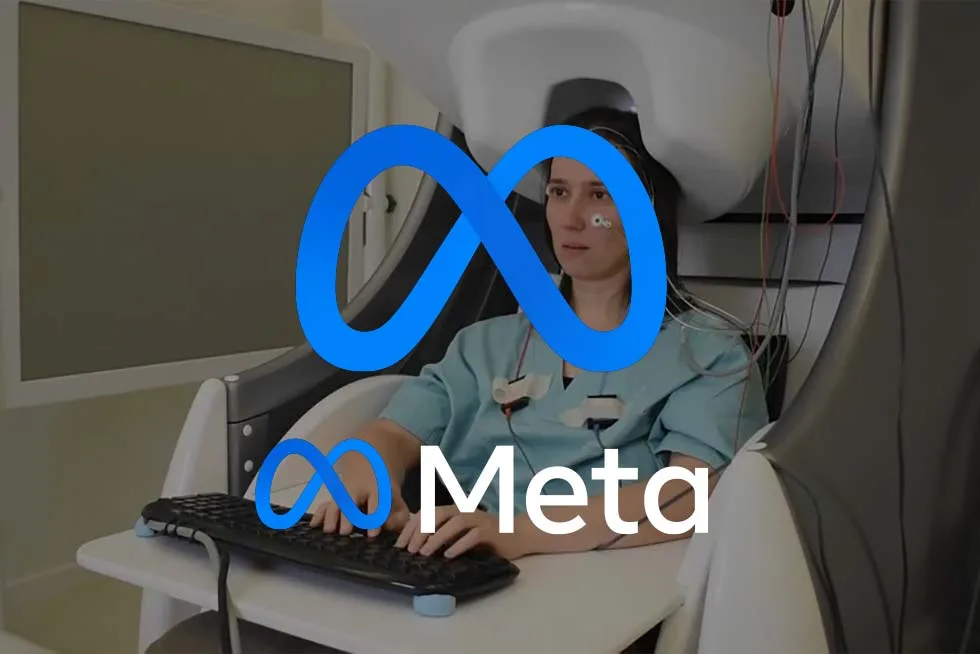
Meta‘s Brain-to-Text AI Technology: Brain2Qwerty
1. Introduction to Brain2Qwerty
Brain-computer interfaces (BCIs) have long been a topic of fascination and research, offering the promise of direct communication between the human brain and external devices. Meta AI’s Brain2Qwerty is a pioneering step in this field, utilizing non-invasive technologies to decode brain activity into text. By interpreting the brain signals of individuals typing on a QWERTY keyboard, Brain2Qwerty provides a potential lifeline for people with speech or motor impairments.
What sets Brain2Qwerty apart is its reliance on non-invasive techniques like MEG and EEG, which eliminate the need for surgical implants. This makes it more accessible and safer compared to invasive BCIs, while still achieving impressive decoding accuracy.
2. How Brain2Qwerty Works
Brain2Qwerty translates brain activity into text through a sophisticated three-stage process. Here’s a closer look at each stage:
- Data Collection:
- Brain2Qwerty uses MEG and EEG to capture brain signals while participants type sentences on a QWERTY keyboard.
- MEG, with its high spatial resolution, provides clearer and more precise brain activity readings, while EEG offers a more portable but less detailed alternative.
- Neural Network Architecture:
- The system processes brain signals using a three-stage neural network:
- Convolutional Module: Extracts spatial and temporal features from raw brain signals.
- Transformer Module: Refines these features and improves contextual understanding of the sequences.
- Language Model Module: A pre-trained character-level language model corrects and refines the output to ensure accuracy and coherence.
- The system processes brain signals using a three-stage neural network:
- Output Decoding:
- The final output is a text representation of the participant’s intended typing, with the system capable of recognizing both correct inputs and typing errors.
3. Key Features of Brain2Qwerty
3.1 Non-Invasive Brain Signal Recording
Brain2Qwerty relies on two non-invasive methods:
- Magnetoencephalography (MEG): Offers high spatial resolution and less susceptibility to signal distortions, making it the preferred method for accurate decoding.
- Electroencephalography (EEG): A more accessible option, though it provides lower resolution and higher error rates compared to MEG.
3.2 Advanced Neural Network Design
The three-stage neural network used by Brain2Qwerty is a key innovation, enabling the system to analyze and interpret complex brain signals with greater accuracy.
3.3 Error Detection and Correction
Unlike many other systems, Brain2Qwerty can detect and correct typing errors by recognizing both motor and cognitive processes involved in typing.
4. Performance Metrics
The performance of Brain2Qwerty varies depending on the recording method used:
- MEG-Based Decoding:
- Achieves a character error rate (CER) of 32% on average, with the best cases reaching as low as 19%.
- EEG-Based Decoding:
- Results in a higher CER of 67%, reflecting the lower resolution and higher noise levels of EEG signals.
These results demonstrate the system’s potential, especially when using MEG technology, to achieve high levels of decoding accuracy.
5. Challenges and Limitations
While Brain2Qwerty is a promising innovation, it faces several challenges:
5.1 Real-Time Implementation
Currently, Brain2Qwerty processes complete sentences rather than individual keystrokes in real time. Bridging this gap is crucial for practical applications.
5.2 Accessibility of MEG Technology
MEG devices are expensive and lack portability, limiting their widespread adoption. EEG could offer a more accessible alternative but at the cost of reduced performance.
5.3 Generalization to Impaired Individuals
Brain2Qwerty has been tested only on healthy volunteers. Further research is needed to evaluate its effectiveness for individuals with speech or motor impairments.
6. Future Directions
The future of Brain2Qwerty holds immense potential. Researchers are working on:
- Enhancing Real-Time Capabilities: Developing models that can process individual keystrokes in real time.
- Improving Accessibility: Exploring ways to make MEG technology more affordable and portable.
- Expanding Applicability: Testing the system with individuals who have speech or motor impairments to assess its real-world impact.
7. FAQ
Q1: What is the difference between MEG and EEG in Brain2Qwerty?
- MEG: Provides higher spatial resolution and is less affected by signal distortions, resulting in better decoding accuracy.
- EEG: Is more portable and affordable but has higher error rates due to lower resolution.
Q2: Can Brain2Qwerty work in real time?
Currently, Brain2Qwerty processes complete sentences rather than individual keystrokes in real time. Researchers are working on improving its real-time capabilities.
Q3: Is Brain2Qwerty suitable for individuals with impairments?
While Brain2Qwerty shows promise, it has been tested only on healthy volunteers. Future studies will focus on its applicability to individuals with speech or motor impairments.
Q4: What is the character error rate (CER) achieved by Brain2Qwerty?
- MEG-based decoding achieves a CER of 32% on average, with the best cases reaching 19%.
- EEG-based decoding has a higher CER of 67%.
8. Conclusion
Meta AI’s Brain2Qwerty represents a significant leap forward in non-invasive brain-computer interfaces. By leveraging advanced neural networks and non-invasive recording methods, it offers a promising solution for individuals with speech or motor impairments. While challenges remain—such as real-time implementation, accessibility, and generalization to impaired users—Brain2Qwerty’s potential is undeniable.
As research continues, Brain2Qwerty could redefine how we communicate, empowering those who are currently unable to express themselves fully. Stay tuned for further advancements in this exciting field!